Teleradiology lets radiologists read scans from anywhere, providing vital overnight coverage for emergency rooms, giving small hospitals quick access to subspecialists, and much more. AI tools now sort urgent images, mark findings, and flag possible problems in seconds, freeing radiologists to focus on tougher calls.
Since these algorithms run on secure cloud systems, experts in different locations can collaborate as if they were sharing the same workstation. This speeds care for trauma, stroke, and cancer patients.
In fact, early studies show that AI can trim report turnaround times by up to 77% and reduce interpretation errors. At the same time, it increases radiologists' satisfaction when it is built directly into normal workflows.
In this article, we will show how these improvements happen, review the data behind them, and outline the challenges that still need solving.
How AI Enhances Teleradiology Workflows
AI changes the radiology workflow at four critical points: intake triage, pixel‑level analysis, remote collaboration, and final sign‑off. Each subsection details one of these touch points and shows how it upgrades speed or quality without displacing the radiologist.
AI Triage and Case Prioritization
Artificial intelligence is transforming how diagnostic radiology practices manage heavy workloads by triaging medical images and prioritizing critical cases. Deep learning algorithms can scan incoming studies (such as CT scans or chest X-rays) and flag those with suspected urgent findings, ensuring radiologists read life-threatening cases first. This automated triage drastically reduces wait times for urgent reads.
For example, an AI system deployed in the U.K. cut the average report turnaround for critical chest X-ray findings from 11.2 days down to 2.7 days. Such workload reduction directly benefits clinical practice. In one meta-analysis of 36 studies, it was found that concurrent AI assistance reduced radiologists’ reading time by ~27% on average. Other important stats from this analysis can be seen below.

By offloading normal exams and highlighting important cases, AI triage prevents backlogs and speeds up care for patients with time-sensitive conditions (e.g., stroke or internal bleeding). In acute stroke, an AI-powered triage system that alerts specialists of large-vessel occlusions can shave 10–11 minutes off time-to-treatment, leading to measurably faster thrombectomies.
The net effect is that radiologists can handle more studies in less time, focusing attention where it matters most.
Automated Detection and Measurement
Deep‑learning “second readers” highlight nodules, fractures, or bleeds and generate volumetric measurements while the radiologist reviews the case, eliminating repetitive mouse clicks. A NIH‑funded evaluation spanning more than 200,000 chest X‑rays showed algorithmic sensitivity equaling or exceeding senior readers for key pathologies.
In oncology follow-up, one tool surfaced 54% of liver metastases that human readers overlooked on routine CT, prompting earlier multidisciplinary discussion. These quantifications, exported as DICOM Structured Reports, feed directly into tumor‑board dashboards and longitudinal registries without extra dictation.
Crucially, radiologists can accept or edit the numbers, maintaining final control while avoiding the drudgery of caliper placement.
Cloud PACS Integration and Real‑Time Collaboration
Web‑native Picture Archiving and Communication System (PACS) platforms now embed AI outputs, including heatmaps, numeric scores, or textual suggestions, directly alongside the pixel data. This means a neuroradiologist in Denver can watch hemorrhage markers update while a trauma surgeon in Montana refreshes the same study from her iPad.
The architecture eliminates file‑transfer bottlenecks and supports synchronous voice or video consults, effectively recreating the group reading room online. Integrated chatbots auto‑compile finding lists, retrieve relevant priors, and even insert guideline citations, shaving precious seconds off every report. Because AI annotations persist in the cloud, subspecialists who pick up a case later see exactly what the first reader saw, eliminating version‑control headaches.
Hospitals, therefore, gain a distributed radiology department without sacrificing the cohesion that drives quality. Here's a visual breakdown of these benefits.
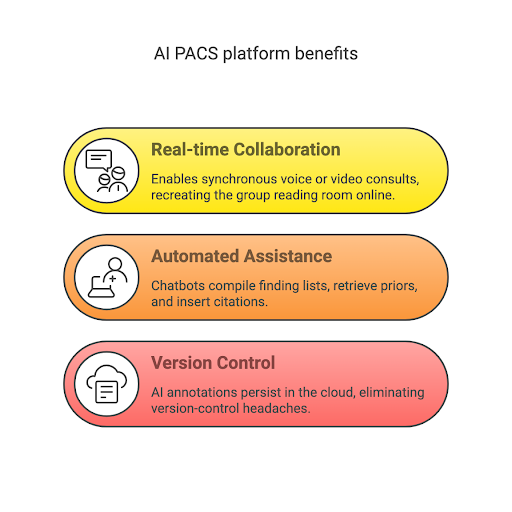
Human Oversight Remains Essential
Every algorithm surfaces probabilities or visual cues, but the radiologist of record still signs the report and assumes legal responsibility. Well‑run services embed validation checkpoints: discrepancies between AI and the reader trigger instant secondary review, while performance dashboards reveal drift before it degrades patient care.
Feedback loops like these resemble teaching interactions with residents, only the learner is silicon, not a human trainee. Over time, institutions report that both the algorithm and the radiologist improve. The AI learns from edge cases, and clinicians learn to question their own blind spots.
The partnership works when each party does what it does best: machines crunch pixels at scale, humans use their skills to synthesize nuance in a clinical context.
Clinical & Operational Benefits of AI in Teleradiology
Here we quantify the measurable gains AI delivers for patients, clinicians, and hospital finances. Each benefit is supported by published data rather than projections or marketing claims.
Shorter Time‑to‑Treatment Saves Lives and Money
Trauma centers using hemorrhage‑detection AI report reductions in emergent craniotomy times, metrics tightly linked to survival and disability. These clinical gains, coupled with downstream cost avoidance, make a compelling value proposition for administrators and insurers alike.
For example, a multicenter stroke system found that AI push‑alerts for large‑vessel occlusion cut door‑to‑neurointervention by 44.13% or around 30 minutes. Because neurons die at roughly two million per minute during an ischemic stroke, that half‑hour corresponds to significant functional recovery on outcome scales.
Financial modeling suggested the workflow would redirect $36.7 million in annual revenue back to community hospitals by reducing unnecessary transfers and compression of length of stay.
“For hospitals, patients, families, and the care team, ideal stroke care involves identifying the patient’s medical needs quickly and transferring the patient only when a higher level of care is deemed necessary to ensure the best outcome. This study showed that ensuring patients are in the right place for stroke care not only improves outcomes but also reduces futile transfers and provides financial benefits.” - Thomas Devlin, MD, Neuroscience Institute Director, Neurologist at CHI Memorial
Productivity Without Extra Headcount
Early‑adopter groups report that turnaround metrics used in hospital service‑level agreements improve immediately, strengthening contract negotiations and referral retention. Because AI also labels straightforward negative exams, radiologists spend a larger share of their effort on complex cases, a change many describe as professionally rewarding.
From a workforce‑planning perspective, AI acts as a force multiplier that combats burnout and postpones the need for expensive recruitment drives. For instance, a national teleradiology vendor recorded an 8.5 % reduction in head CT reporting time across 18,550 studies after deploying an AI assistant. Scaled across a fiscal year, that equates to thousands of radiologist hours.
Higher Diagnostic Consistency and Safety
AI algorithms don't get tired, meaning their accuracy stays consistent. By highlighting issues like missed tumors, hidden fractures, or inaccurately measured aneurysms, AI helps radiologists maintain high-quality, consistent diagnoses.
It also ensures that all medical reports include standardized measurements, like tumor size and growth rates, making it easier for hospitals to collaborate and compare data effectively. When errors do occur, the clear, structured information provided by AI simplifies identifying and addressing the cause, speeding up necessary training.
Because of these benefits, malpractice insurance companies are starting to offer reduced premiums for medical practices that use AI to double-check diagnoses, as this approach has been linked to fewer insurance claims.
Foundations for Precision Medicine
Radiomics pipelines convert pixel patterns into quantitative biomarkers that correlate with tumor genotype and therapeutic response; early pilots now attach malignancy probabilities or recurrence scores to routine CT impressions.
Clinicians then combine those scores with circulating‑tumor‑DNA assays or electronic‑health‑record phenotypes to create individualized treatment protocols. Researchers are testing whether radiomic signatures can predict immunotherapy toxicity before cytokine storms occur, a possibility that could spare patients intensive‑care admissions.
Although many studies remain investigational, regulators have already cleared limited prognostic algorithms, signaling a regulatory appetite for evidence‑based personalization. Radiologists, once stewards of purely anatomic data, are thus evolving into curators of multimodal biomarkers that drive precision oncology.
AI in Teleradiology Examples: Common Use‑Cases & Success Stories
Operational pilots reveal where AI already pays off, from emergent to longitudinal imaging.
Mass‑scale Intracranial Hemorrhage Screening
A U.S. service processed 61,704 head CTs with Avicenna.ai’s CINA algorithm, achieving 86 % sensitivity and 93 % accuracy compared with fellowship‑trained neuroradiologists. Positive detections triggered smartphone alerts.
These alerts often reached surgeons before technologists finished sending reformatted series, shaving crucial minutes off emergency craniotomy decisions. Institutions reported that the AI never slept or stepped away, so zero‑miss performance during shift changes became realistic rather than aspirational. Because ground‑truth labels were continuously updated, the algorithm’s sensitivity rose two percentage points over the first six months, illustrating the value of real‑world feedback.
Chest‑X‑Ray Triage During Respiratory‑Virus Surges
At the height of a winter RSV–COVID overlap, an emergency‑department study ran around 20,000 portable films through Lunit INSIGHT CXR and achieved 99% specificity for urgent abnormalities. Cases labeled “normal” were safely deferred to daylight hours, freeing night staff to tackle complex ICU and trauma work without extending shifts.
By clustering flagged findings like pneumothorax, line malposition, or airway foreign body at the top of the list, the hospital cut average life‑critical report times from 42 to 14 minutes. Radiology leadership credits the software with preventing staffing‑related backlogs that plagued peer institutions during the same surge.
“This validation is crucial to overcome the limitations of previous retrospective studies and to address concerns about the applicability of AI across varied patient demographics and clinical scenarios.” - Srinath Sridharan, PhD, of Changi General Hospital
Oncology Follow‑Up Automation
In metastatic liver surveillance, deep‑learning software that aligns serial MRIs flagged new 2–3 mm nodules weeks before they crossed RECIST thresholds. Oncologists capitalized by escalating systemic therapy sooner, a change now being tracked prospectively as a surrogate for progression‑free survival.
The same platform auto‑populates standardized tables in the radiology report, sparing physicians from manual spreadsheet maintenance. Patients appreciate the consistency: regardless of which radiologist reads the study, the numeric response metrics look identical, building confidence in longitudinal care. Pharmaceutical sponsors are exploring algorithmic response assessment to streamline eligibility screens in decentralized clinical‑trial designs.
CMS‑Funded Stroke Triage
Viz.ai’s large‑vessel‑occlusion detector became the first radiology‑AI to earn a Medicare New Technology Add‑on Payment (NTAP), validating financial as well as clinical impact. Hospitals that adopted it report return‑on‑investment within the NTAP window, citing shorter length of stay and higher thrombectomy capture. Because CMS renewed the add‑on when early data expired, stakeholders view the decision as a signal that the agency values outcomes as much as cost control.
NTAP success has inspired vendors in pulmonary embolism and gastrointestinal bleeding to pursue similar reimbursement pathways. If even a fraction succeed, AI might soon be table‑stakes for time‑sensitive imaging diagnoses.
Barriers to Adoption
Privacy, tech debt, and staffing fears still loom. These challenges must be resolved for AI to scale responsibly.
Data Privacy and Ransomware Risk
Medical images are coveted by cybercriminals; one Northeast practice paid $350,000 after 300,000 studies leaked from an unsecured PACS. Separately, an attacker exfiltrated 866,000 patient records from a Carolina chain. Because AI servers often sit alongside PACS or live in the cloud, they introduce new attack surfaces if not hardened and patched vigilantly.
Regulators now expect business‑associate agreements, HITRUST certification, and end‑to‑end encryption before approving remote‑read vendors, raising compliance overhead. Best practice dictates de‑identifying images used for model training and segmenting clinical networks so malware cannot hopscotch from modality to data lake.
Workforce Adaptation and Culture Change
Radiologists who help select and tune algorithms report higher trust and smoother workflows than those handed a black box on go‑live day.
According to a recent study, radiologists modified AI algorithm outputs in 93% of cases, and these human adjustments led to improved diagnostic outcomes 60% of the time. These findings highlight why involving radiologists in the AI integration process is essential for enhancing diagnostic accuracy and overall workflow efficiency.
Many groups now market AI‑assisted shifts as a burnout antidote, emphasizing the reduction in repetitive measurements and the opportunity to focus on consultative work. Residency curricula are evolving to include prompt engineering, bias detection, and basic data science so tomorrow’s doctors feel fluent rather than threatened.
Ultimately, AI may alleviate rather than exacerbate the projected radiologist shortage by letting existing staff cover more ground without sacrificing accuracy.
Regulatory & Legal Landscape
Rules and incentives are catching up to the technology. The next four items show how regulators and payers are laying the groundwork for responsible, sustainable AI adoption.
FDA Oversight Evolves for Adaptive Models
Traditional 510(k) pathways assume static code, yet AI may improve weekly or even daily. The 2024 Predetermined Change Control Plan (PCCP) guidance allows vendors to pre‑specify data, validation, and rollback criteria for post‑market updates, enabling safe iteration without resubmitting a new clearance.
Companies that secure PCCP approval can patch performance gaps within weeks rather than years. Because updates remain locked to predefined parameters, regulators retain visibility, and clinicians retain confidence that accuracy will not degrade unexpectedly. Early adopters view PCCP as the missing link between rigid device regulation and the agile software lifecycle that learning models require.
Reimbursement Inches Forward
CMS’s Viz.ai NTAP opened the door for other inpatient algorithms, while outpatient Category III CPT codes now track the work of quantitative imaging AI even if payment remains optional. Private insurers and radiology benefit managers are beginning to ask whether AI was used for high‑stakes pathways such as mammography double‑reading.
Hospitals pursuing value‑based‑care contracts see AI as a hedge; if algorithms reduce downstream complications, shared‑savings bonuses flow back to the imaging department. Some early adopters bake AI fees into professional‑service contracts, arguing that improved throughput lets them read more studies at the same blended rate. Clearer billing guidance would accelerate adoption, but the financial trend line is already pointing in AI’s favor.
Professional Societies Codify Best Practices
The 2024 guidelines issued by leading radiology organizations, including the American College of Radiology (ACR) and Radiological Society of North America (RSNA), recommend that healthcare facilities create dedicated committees to oversee AI use, establish clear checklists when purchasing AI systems, and regularly audit AI accuracy.
Importantly, these guidelines emphasize that radiologists remain responsible for diagnoses. Since courts often use such professional guidelines to determine the standard of medical care, institutions that do not follow them risk increased legal trouble.

Additionally, the guidelines encourage hospitals to involve not just radiologists but also IT staff, compliance experts, and other healthcare professionals in AI decision-making, promoting teamwork rather than isolating AI as a purely technical innovation.
Ultimately, the guidelines position AI as a supportive tool rather than an independent decision-maker, aligning its use with patient safety and ethical standards. As legal cases involving AI become more common, following these expert recommendations will likely become as important as having FDA approval for protecting healthcare providers in court.
Future Trends Shaping AI‑Driven Teleradiology
Technology and policy continue to evolve. The innovations below point toward faster, more connected, and more personalized imaging in the next decade.
Generative AI Copilots Enter the Reading Room
Large‑language models such as GPT‑4 equaled radiologists at spotting report inconsistencies in a blinded trial. Another pilot used ChatGPT to generate plain‑language summaries that patients preferred to the original report, cutting phone‑call follow‑ups by 22%.
Vendors are now fine‑tuning LLMs on de‑identified report corpora so the models can auto‑populate finding sections, cross‑reference guidelines, or flag incomplete clinical indications. Because hallucination remains a documented risk, early deployments keep the radiologist in the loop, highlighting AI‑added text for quick acceptance or revision. If reliability continues to improve, copilots could eventually draft the entire impression, letting physicians focus on value‑added consults with care teams.
Federated Learning Democratizes Algorithm Training
By sending encrypted weight updates instead of images, hospitals from Alaska to Alabama can collaboratively improve models without violating HIPAA or GDPR.
Early federated networks boosted pneumothorax‑detection AUC by six percentage points over single‑site training, proving the value of geographic diversity in data. Because local data never leaves the firewall, providers sidestep onerous data‑use agreements while still influencing algorithm performance on their patient mix.
Several PACS vendors now bundle federated frameworks, so participation requires little more than a checkbox in the admin panel. The approach could accelerate equitable accuracy across demographic and device variations that single‑institution datasets miss.
Edge AI Bridges Bandwidth Gaps
GPU‑powered boxes small enough to sit on a modality cart can run stroke or pulmonary‑embolism detection locally, returning results before the technologist exits the scanner room.
When satellite clinics reconnect to the cloud, model updates and analytics sync automatically, preserving a unified learning loop while guaranteeing sub‑minute latency on site Edge inference also alleviates privacy concerns because raw images never leave the premises, simplifying cross‑border compliance.
New hardware accelerators consume less than 50 watts yet process 3D CT volumes in under 20 seconds, opening AI to ambulatory and mobile imaging units. As 5G and fiber reach rural America, the line between edge and cloud will blur, creating a seamless tiered compute fabric for radiology.
Toward a Borderless Imaging Ecosystem
Next‑generation JPEG‑XS and AI‑optimized streaming shrink a full CT angiogram to tens of megabytes, enabling near‑real‑time consults between radiologists in opposite hemispheres. Smart routing engines assign each study to the first awake neuroradiologist with documented excellence on that modality, balancing workloads across time zones.
Large language models then stitch imaging, labs, and genomics into a longitudinal patient timeline, ensuring every consultant sees the same curated story. Regulators will have to address licensure and liability, but the technical scaffolding for a 24/7 global reading network already exists. For patients, that means faster results and access to niche expertise regardless of geography, insurance network, or hospital size.
AI is Here to Stay in Teleradiology
Evidence to date shows that AI accelerates teleradiology workflows, boosts diagnostic quality, and strengthens the business case for remote imaging services. Regulatory innovations such as the PCCP, early reimbursement wins like NTAP, and maturing interoperability standards are removing historical roadblocks at the same pace that GPU performance and cloud bandwidth rise.
Generative copilots, federated learning, and edge hardware hint at an ecosystem where radiologists orchestrate information rather than hunt pixels, reserving their cognitive bandwidth for complex decision‑making and patient communication. The profession’s core clinical judgment and patient advocacy remain irreplaceable, yet its toolbox is expanding rapidly. Organizations that pilot, measure, and refine AI today will be best positioned to deliver faster, fairer, and more personalized imaging tomorrow.
Frequently Asked Questions
Will AI replace radiologists or augment them?
AI is designed to augment rather than replace radiologists. It automates repetitive tasks and enhances diagnostic accuracy, allowing radiologists to focus on complex cases and patient care.
What ROI can imaging groups expect from AI tools?
Imaging groups often see significant returns on investment through increased efficiency, reduced diagnostic errors, and improved patient throughput. Many practices report measurable cost savings and higher productivity shortly after implementation.
How secure is AI-enabled teleradiology?
AI-enabled teleradiology platforms adhere to stringent security standards to protect patient data. Reputable solutions comply with HIPAA guidelines, use encrypted communication channels, and employ robust cybersecurity measures.
What are the benefits of AI in teleradiology?
AI enhances teleradiology by improving diagnostic accuracy, reducing report turnaround times, and minimizing radiologist fatigue. It also helps standardize interpretations, reducing variability and improving overall patient outcomes.
Which certifications or clearances matter when buying an AI tool?
When purchasing AI tools, look for FDA clearance, CE marking (for European markets), and compliance with healthcare data standards such as HIPAA. These certifications ensure reliability, safety, and effectiveness in clinical practice.